Generally speaking there are two ways you can do this – through descriptive statistics and inferential statistics.
Types of Statistical Analysis
Descriptive statistics are just like they sound a way of describing the data, this can typically be through the use of reporting percentages or using tables and graphs to illustrate your data. Inferential statistics are to do with exploring statistical analysis to check for statistical significance.
Broadly there are two different type of inferential tests that we can undertake with our quantitative data. We can assess for a relationship within the data or we can look for differences between the data.
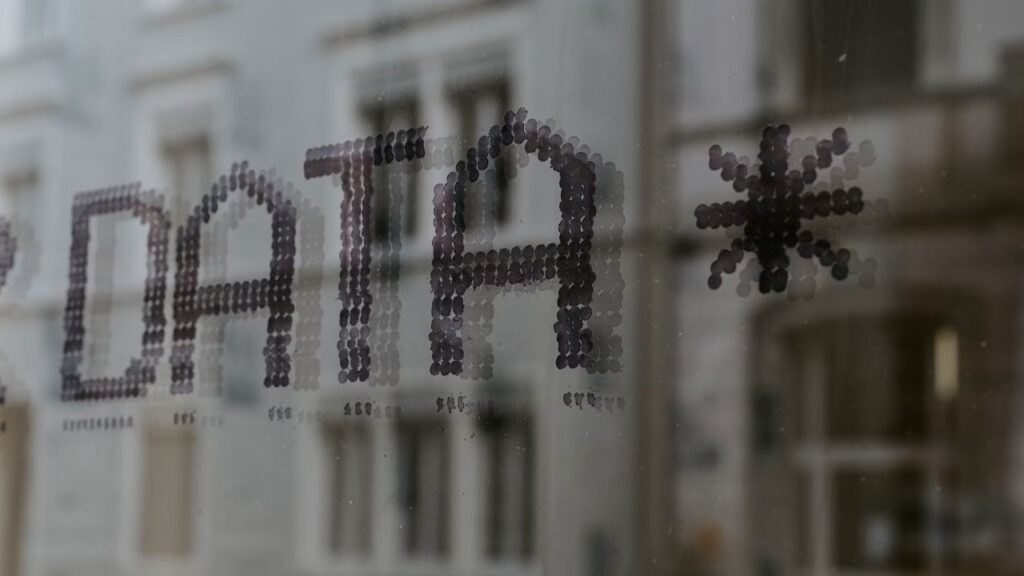
Types of Inferential Tests
For example a test of relationship may well employ a correlational analysis. For example I might want to test if there is a relationship between number of minutes per a week that someone exercises and their resting heart rate.
Using a survey I gather this information on these two variables from a sample of people. I then have what is known as bivariate data (this really just means two sets of data).
With this data I can undertake a correlation analysis, this analysis will show me if there is a relationship between the two variables and also the strength and direction of that relationship.
It may be that I find that the more you exercise the lower your resting heart rate, this would be termed as a negative correlation as when one variable increases the other decreases. Our analysis will also tell us if this relationship is statistically significant (is our result likely to be due to chance alone).
Alternatively, we may be looking for a difference within our data. So, for example I have one group of people and I measure their resting heart rate, I then put them on a two week exercise programme and then measure their resting heart rate for a second time.
I would predict that their resting heart rate would have gone down after the exercise intervention. I would then test if there was a statistically significant difference between the before and after measures.
This would show if any difference was likely to be due to chance alone.
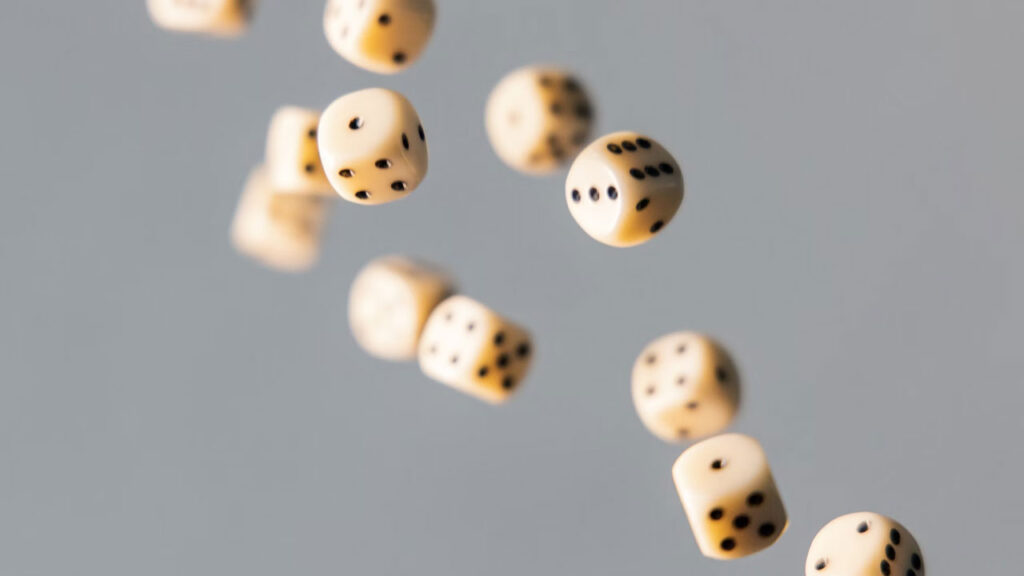
The Role of Luck in Statistical Analysis
Underlying our data analysis, especially any inferential analysis, is the desire to check if any relationships or differences we observe are just down to blind chance/luck.
For example we need to be pretty sure that our observed changes or differences are due to what we have manipulated or changed, as we want to reduce the probability that we can got our conclusions wrong.
Technically within statistical test the chance of us being wrong, and misattributing a result that is just due to chance alone, is called the alpha level, and is most cases this is at the 5% or 0.05 level.
This means that even when we do find statistically significant results, there is always a chance (although it may be small) that what we have observed is through chance alone.
This is one of the reasons that we never claim to have proven a claim or hypothesis, we can only say whether or not our data analysis supports our ideas or theories.
If you would like more help or guidence on this topic please feel free to get in touch with me to discuss your specific needs and I’ll be happy to help.